Quote: “Artificial intelligence would be the ultimate version of Google. The ultimate search engine that would understand everything on the web. It would understand exactly what you wanted, and it would give you the right thing. We’re nowhere near doing that now. However, we can get incrementally closer to that, and that is basically what we work on.” — Larry Page (Co-founder of Google)
AI (Artificial Intelligence) is dynamically changing the digital world and business operations. In pairs with ML (Machine Learning), it gives the maximum boost to any business’ credibility. AI/ML applications account for 20% growth in the digital world [Since 2020]. So, hosting these applications for businesses through GPU cloud servers has become the trending prerequisite nowadays after the pandemic.
According to reports, 48% of businesses leverage machine learning, data analysis, or AI to gain a competitive edge. Now, if you want to employ these advancements, bet on the robust IT infrastructures that the GPU cloud server offers.
In this guide, we will explain why a GPU cloud server is the right fit for hosting AI/ML applications. Delve deeper into the article without further delay.
Table Of Content
What is Cloud GPU?
GPU stands for Graphic Processing Unit, a high-performance processor that uses cloud infrastructure to process complicated operations. Users access cloud GPUs through cloud service providers, like MilesWeb, Google Cloud, and Amazon Web Services (AWS), without the physical hardware requirement.
Cloud GPUs are used for different tasks including AI, ML, video editing, and scientific computing. Cloud GPUs can be used to play high-quality games on a smartphone, tablet, or laptop without the need for expensive hardware.
Whether to use a cloud GPU or purchase your own GPU hardware depends on your needs and budget. If you need complete control over your hardware and software stack or have a high utilization rate for GPUs, then buying your own GPU hardware might be the best option.
How GPUs facilitate AI and ML Workloads?
GPUs are multiple microprocessors installed in the system that perform tasks like graphic processing and simultaneous computing by using the parallel processing capabilities and higher memory bandwidth.
Intensive computing tasks like gaming, 3D imaging, video editing, crypto mining, AI, and Machine Learning require dense computations. GPUs have dense computations that streamline the processes to make sequential operations smoother at very low latency. GPUs have powerful CPU cores that can easily process volumes of workloads.
AI/ML and deep learning are some of the advancements that needed a strong hardware architecture to process volumes of data simultaneously. This is where the GPU hosting steps in. Initially, GPUs were used for gaming and other graphics-intensive projects. However as their parallel processing capabilities become clearer, they became an ideal match for deep learning applications.
How GPUs Work?
GPUs facilitate several technical operations to work simultaneously as a large number of cores work together. The parallel processing architecture enables the GPUs to manage the tasks that the CPUs would manage in a longer time.
Just imagine when a task can be broken down into smaller and thousands of parts and independent steps. By distributing these steps across many of the cores in a GPU, we can have all these steps computed in parallel. Compared to CPUs, the GPU’s multi-process capability provides it other stern advantages, notably in image and video processing, scientific simulations, and machine learning, where large datasets and the complexity of algorithms are required.
Benefits of Cloud GPUs in Hosting AI Applications
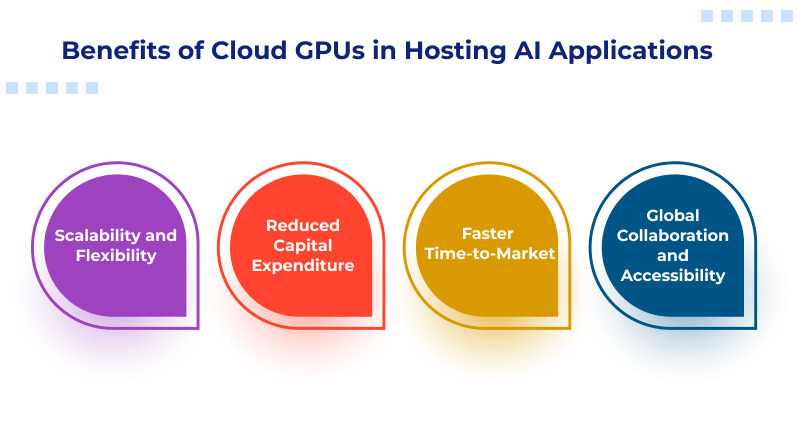
1. Scalability and Flexibility
The scalability of cloud GPUs for AI workloads is just huge. It lets businesses quickly scale their computing up and down automatically based on demand, using cloud infrastructure. This flexibility lets AI developers work with powerful GPUs for intensive resource tasks like training large deep learning models without the upfront cost of expensive hardware. This on-demand model also eliminates cost, as companies pay only for what they use and thus serves as a cost-effective solution to projects with varying computational requirements.
Secondly, the resources can be scaled easily, which means AI teams can try out larger datasets, and more complex models, without worrying if they would run out of resources. Such flexibility is necessary in machine learning projects, which often involve a bunch of iterations or require large amounts of data to work on. Developers no longer have to wait for new equipment to be installed or upgraded — cloud GPU services make it easy to leverage the most recent equipment without having to buy new hardware on their own.
2. Reduced Capital Expenditure
One of the significant benefits of cloud GPUs is the reduction in capital expenditures. This is usually an expensive upfront investment by any traditional AI infrastructure: many high-performance GPUs, massive server farms, and tremendous data centers. Companies lacking in this budget find relief with cloud GPUs. Companies like AWS, Google Cloud, and Microsoft Azure extend the services of their respective top-shelf GPUs on a pay-as-you-go basis without forcing any big capital outlay.
It’s then possible for organizations to concentrate on optimizing their AI models, rather than managing the hardware. This means saving costs on hardware maintenance while at the same time reducing the dependency on specialized IT personnel in handling infrastructure. It comes with no upfront and no long-term commitments so that companies can use the available budget for innovation and development rather than for acquiring and maintaining physical resources.
3. Faster Time-to-Market
Cloud GPUs enable AI teams to develop much faster. As long as powerful computing resources can be provided on demand, the time required to train and fine-tune the machine learning models is reduced drastically. With this faster processing power, AI developers can easily experiment with numerous algorithms and adjust their hyperparameters, without the wait for the hours or days required for hardware provisioning. The speed of cloud GPUs can thus reduce time-to-market, allowing businesses to launch AI-driven products and solutions faster than ever before.
What is more, cloud services, mostly have pre-configured AI-friendly environments that are quite less to set up than an equivalent in-house version, and thus less likely to keep developers busy while getting down to the brass tacks of core development work. Speed of prototyping and deploying models is something a very competitive advantage, as fast as the AI field and where all speed is of an advantage.
4. Global Collaboration and Accessibility
Cloud GPUs foster greater collaboration among AI teams across different geographical locations. Cloud-based infrastructure can be reached from any place with the internet so that developers and researchers from around the world can work on the same AI projects without any constraints of hardware. This global accessibility is a big benefit that allows for accelerated innovation as AI professionals from numerous backgrounds and specialties can be a part of a project in real-time, adding to the quality of the models being built.
Additionally, specialized GPUs that may be hard to acquire locally for ordinary AI companies or researchers can be easily obtained by AI companies or researchers. Access to a good variety of GPU types is the norm for cloud GPU services, optimized for different jobs like training, inference, and data processing. By democratizing high-performance computing, this allows smaller organizations or startups to compete on a worldwide basis, eliminating the winning ability to compete in the AI industry.
In conclusion, Cloud GPUs have become an indispensable tool in the development of modern AI, offering a range of benefits that conventional hardware simply cannot match. From providing unparalleled scalability and flexibility to reducing capital expenditure and accelerating time to market, cloud-based GPU solutions empower businesses and developers to push the boundaries of innovation.
High-performance computing resources are now more within the reach of any organizational size without the requirement to make a significant upfront investment using AI. Furthermore, cloud GPUs help create more powerful, effective AI models because they provide world access and collaboration. Moving forward, AI will surely only be a cornerstone in establishing the future as it comes, and breakthroughs can now be achieved, considered previously unimaginable, using Cloud GPUs.
FAQs
How do Cloud GPUs differ from traditional GPUs?
Cloud GPUs are virtualized and accessed remotely via cloud services, allowing on-demand access without physical hardware. Traditional GPUs require upfront investment and are limited to the local system’s capabilities. Cloud GPUs offer scalability, flexibility, and the latest hardware, which is not possible with fixed traditional GPUs. They also eliminate the need for maintenance and hardware management.
Why are Cloud GPUs important for AI and ML?
Cloud GPUs provide the processing power needed to handle the computational demands of AI and ML workloads. They allow for faster training of models, particularly in deep learning, which requires intensive parallel processing. With cloud scalability, users can adjust resources based on workload needs, making it cost-effective. Cloud GPUs also ensure access to the latest, high-performance hardware for cutting-edge AI research.
How do I choose the right Cloud GPU for my AI/ML workload?
Choose a Cloud GPU based on your workload’s complexity, dataset size, and task requirements. For deep learning, look for GPUs with high memory and processing power, like NVIDIA A100 or V100. For lighter tasks, options like NVIDIA T4 or A10 may be sufficient. Consider pricing, GPU capabilities, and compatibility with your AI frameworks to make the best choice.
How can I monitor and manage Cloud GPU resources?
You can monitor Cloud GPU usage using tools like AWS CloudWatch, Google Cloud AI Platform, or Azure Monitor. These tools provide insights into GPU utilization, memory usage, and processing performance. Managing resources involves scaling GPUs up or down based on demand and turning off idle resources to save costs. Alerts can also help track usage and optimize GPU performance.