Having a clear understanding of what is GPU in cloud computing is necessary because businesses are seeking to improve their workloads. By using services such as free cloud computing and NVIDIA GPU cloud computing, organizations can tap into high-performing resources without having to make substantial financial investments.
Also, owing to the parallel processing power of cloud GPU services have triggered a revolution in performance-intensive computing, offering significant speed improvements for multiple operations, such as AI (artificial intelligence), scientific modeling, and image rendering.
Cloud computing environments have quickly accepted these developments, which makes GPU on cloud resources easily accessible to all businesses starting from healthcare and manufacturing to finance and entertainment.
Moreover, what is even more intriguing is the anticipated growth of the GPU as a Service market, which is predicted to rise from $3.16 billion in 2023 to a remarkable $25.53 billion by 2030. Such a transformation pinpoints the growing need for modern data processing capability.
Additionally, by taking advantage of GPU on cloud services, businesses can effectively speed up growing resource-intensive processes. The scalability and versatility provided by these powerful cloud computing services streamline the use of GPU power by helping businesses stay competitive in an existing data-centric world.
Table Of Content
What is GPU in Cloud Computing? And How Does it Function?
GPU in cloud computing refers to the process of integrating GPUs in cloud infrastructure in order to improve and boost diverse computing processes. Contrary to the conventional single CPUs, GPUs in cloud are developed for managing parallel processing, which is a technique used to break down huge, and complicated workloads into smaller tasks that can be finished simultaneously.
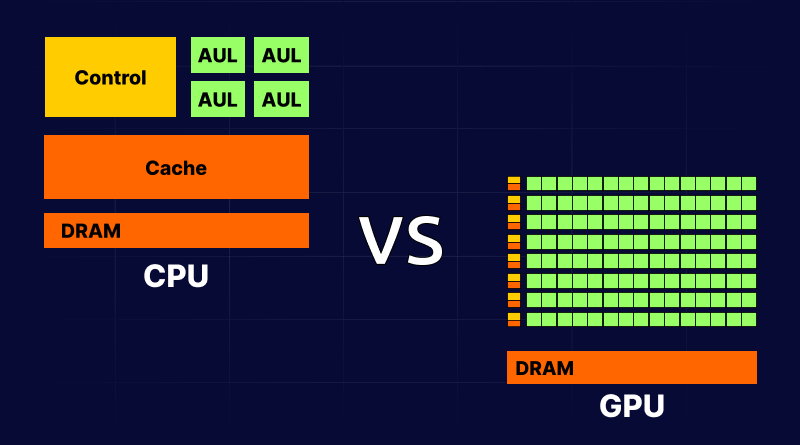
This approach is specifically beneficial for workloads such as ML, AI, and big data analytics, where the GPU’s parallel processing capabilities can:
- Lower the time required to execute a program
- Accelerate the process of code execution
- Increase the speed and performance of big data management
Now that we’ve got a clear understanding of what is GPU in cloud computing the next thing to understand is CPU vs GPU and how it actually operates. GPUs are linked together in a data center with the help of a high-speed networking system.
In order to enhance the workflow distribution, task-scheduling algorithms, and load-balancing strategies are used after making sure that data has been moved from storage to GPU cloud servers, thus, drastically increasing the computational speed. Once the tasks are finished, the outcomes are sent back to the users.
Suggested Reading: What is a Cloud GPU? Understanding the Benefits and Major Cloud GPU Providers
Core Benefits of GPU in Cloud Computing Technology
GPU in cloud technology can be considered a financial model based on ongoing operational costs or as a capital investment framework. It is specifically beneficial for businesses that are looking for HPC (high-performance computing) capabilities in a data center or via an external cloud service provider.
The core benefits of using GPU in cloud consist of improved resource utilization and versatility, increased processing power, faster deployments, rapid experimentation and innovation, and availability across the world.
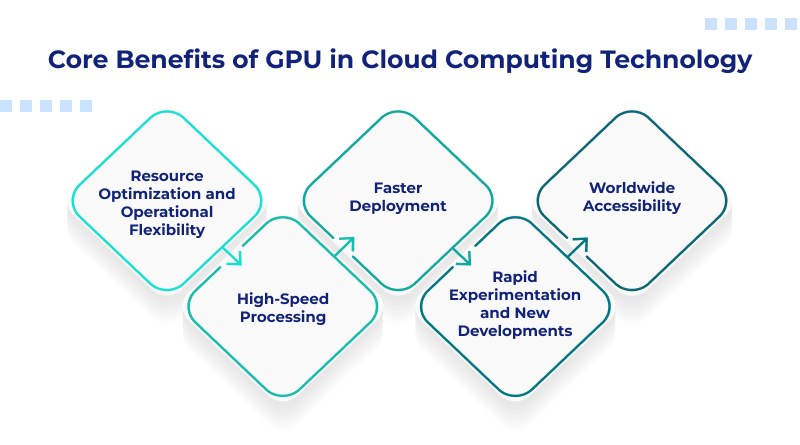
1. Resource Optimization and Operational Flexibility
Because of the flexibility of easy integration with a shared, pay-as-you-go cloud framework, customers can adapt GPU resources in real time in order to meet their particular requirements. It means that at times of increased computational need, such as when executing complicated simulations or training machine learning models, users can effectively scale up the number of virtual GPUs.
When there’s less demand, resources can be minimized to maximize cloud cost efficiency, thereby users can avoid unwanted expenditure on dormant resources. This scalability in resource distribution helps organizations to efficiently adapt to fluctuating needs, which makes sure that the computational capabilities are closely aligned with the requirements of particular tasks.
2. High-Speed Processing
High-speed processing is a prominent benefit due to its exceptional processing capabilities and efficiency. The parallel processing power of the GPU in cloud facilitates higher returns and rapid execution of several tasks in parallel, thereby enhancing the speed of artificial intelligence and analytics workloads.
The capability to swiftly add or reduce GPU resources within the cloud as required, also facilitates customers to meet varying operational needs, making sure that enhanced processing power is readily accessible whenever it is required.
3. Faster Deployment
Configuring and integrating GPU resources in the cloud is a simple process. Customers can instantly set up virtual GPUs and start their workloads without any delays related to purchasing and setting up physical hardware.
4. Rapid Experimentation and New Developments
Within a shared GPU cloud computing platform, users have sufficient flexibility to investigate and experiment with modern techniques and methods, without any restrictions related to dedicated hardware.
By offering an environment for experimenting and refining computational frameworks and algorithms, it enables businesses to stay competitive by accelerating quicker innovation and deployment processes.
5. Worldwide Accessibility
Because of the cloud’s inherent capabilities, resources are accessible from any location that has an internet connection based on different elements like:
- Standards for Compliance
- In-house safety constraints
This allows for collaboration between various teams distributed across multiple geographical locations, enabling them to work collectively on challenging tasks.
Leading Industries Utilizing GPU Cloud Computing
Multiple industries are embracing the power of free GPU cloud computing to fasten their resource-intensive workloads and speed up operations. In the case of AI and ML, GPUs significantly minimize training time for complicated workloads, empowering innovations in natural language processing and visual recognition technologies.
While the healthcare industry is dependent on GPU in cloud computing resources as it supports advancements in medical imaging, diagnostics, drug discovery, and providing more precise results.
Other industries like media and entertainment make use of NVIDIA GPU cloud computing for image rendering, animation, and dynamic video processing, thus, refining production standards and operational efficiency.
In the finance industry, GPU cloud computing helps with real-time data analytics and predictive modeling, whereas, scientific research takes advantage of their processing power in effectively managing simulations and data-heavy analyses.
Challenges of GPU in Cloud Computing
As seen earlier, there are significant advantages to utilizing GPU in cloud computing, as is the case with most things, there are certain potential barriers as well. To effectively resolve these challenges, a well-thought-out strategy is required blending technical skills, careful planning, and an in-depth understanding of internal needs and industrial standards.
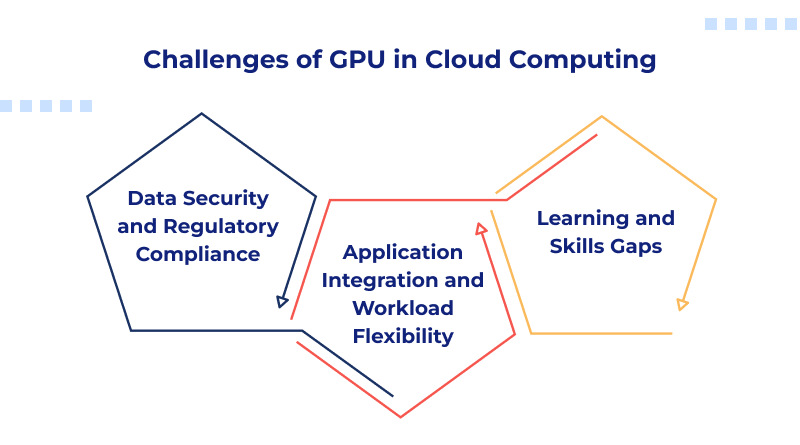
1. Data Security and Regulatory Compliance
A wide array of data security laws and regulations, together with specific industry regulations, can make it challenging to assure compliance with regional data residency needs when transferring sensitive information.
Additionally, the resource-intensive tasks that usually utilize GPU cloud computing normally contain sensitive information. To resolve this, deploying powerful safety protocols against malicious threats, encryption, and access controls are essential, where selecting a dedicated environment is the best option in multiple instances.
Furthermore, it is important to completely grasp data privacy and compliance standards, and also being aware of the shared responsibility structure between your cloud provider and organization is crucial for successfully managing data security and compliance issues.
2. Application Integration and Workload Flexibility
Regrettably, even if each application, software, and library is compatible with GPU cloud architecture, not all will be able to derive benefits from their capabilities. In order to reap the benefits of GPUs on cloud, legacy apps or software might be required to go through modifications in order to function correctly in a cloud setting.
It could also be challenging to guarantee that, GPU-accelerated operations are easily portable between various cloud platforms or on-site systems. Therefore, carrying out necessary tests to evaluate their portability is equally crucial.
3. Learning and Skills Gaps
Changing to a new system might involve a significant learning curve for IT personnel who aren’t familiar with particular programming frameworks and optimization technologies and adjusting to current software to effectively take advantage of GPU capabilities can also be difficult, demanding expertise in parallel processing.
In addition to that, appropriately handling GPU resources such as performance monitoring, software update handling, and resolving problems could be challenging. Therefore, for the optimum use of GPU in cloud, it’s crucial for businesses to consider investing in learning and training programs or look for talent, which can be from third-party providers or recruiting new talent internally, to fill any skill gaps related to the cloud technology.
GPU in Cloud Computing: Future Trends
GPU in cloud computing is ready for tremendous improvements in years ahead, fueled by the growing need for modern processing capabilities in a wide array of industries. One of the most noteworthy trends is embedding AI and ML technologies within free GPU cloud computing services, which significantly improves data analysis and decision-making capabilities.
Apart from that, the advent of edge computing will continue to enhance real-time data processing, by positioning GPUs nearer to the data source thereby resulting in better latency. As businesses search for affordable solutions, pursuing GPU as a Service model is expected to grow, granting users to harness robust computational resources without the burden of substantial initial expenses. Ultimately the future of GPU in cloud computing holds great potential for improved performance and advancements.
Currently, organizations of all sizes from startups to large enterprises can break through previously unmanageable complexities and can accelerate advancements by tapping into the potential of GPU in cloud.
If you’re dealing with a huge dataset for training computer vision models, turn to GPU cloud computing for the solution. Do you want to develop numerous design prototypes? Accelerated cloud workstations are here to assist you.
It’s truly exhilarating to see the expansion of free GPU cloud computing technology – the prices are falling and hardware is evolving continuously. The best part is – we’re just starting to explore the huge potential of accelerated computing power in the cloud setting.
MilesWeb’s next-level NVIDIA-powered GPU cloud computing helps simplify computational management and is perfect for all industries. You can achieve unmatched performance across all intensive tasks using their guaranteed resources, world-class hardware, and processors. With the option to choose from various NVIDIA-GPU-ready cloud solutions, you can meet all your needs.
Get started with MilesWeb now and harness the full potential of AI, HPC, and graphics without overspending.
FAQs
What are the key benefits of GPU on cloud for businesses and individuals?
GPU in cloud provides flexibility, allowing businesses to access HPC capabilities via scalable resources. Other advantages include improved performance, faster processing, speedy deployment, and worldwide availability. Using the pay-as-you-go model, you can easily scale up cloud resources during peak hours.
In what ways does GPU in cloud speed up AI and ML processes?
Cloud GPUs speed up data processing activities such as filtering, sorting, and aggregating huge datasets. It is important in exploratory data analytics, where data is analyzed to uncover valuable insights and trends within data.
How do I decide which GPU in cloud best matches my requirements?
While price matters, it isn’t the only element to consider. Remember to evaluate other crucial elements, such as GPU types and configurations that fulfill your needs, and resource allocation, such as RAM, storage, and network bandwidth.
How can I optimize the performance of my cloud GPU?
To improve GPU cloud performance, you should choose the ideal GPU instance, speed up GPU drivers and associated software, effectively handle memory utilization, increase throughput using parallel and batch processing, simplify data transfer methods, and consistently monitor and refine performance parameters.